Introduction
In today’s fast-paced digital landscape, businesses face the challenge of staying ahead of the curve and anticipating trends before they occur. Predictive analytics emerges as a powerful tool to address this challenge, offering insights that enable organizations to make informed decisions and capitalize on future opportunities.
Understanding Predictive Analytics
Predictive analytics involves the use of data, statistical algorithms, and machine learning techniques to forecast future outcomes based on historical data. By analyzing patterns and trends, predictive analytics enables businesses to anticipate changes in market conditions, customer behavior, and other factors that impact business performance.
Data Collection and Preparation
The success of predictive analytics depends on the quality of the data used for analysis. Therefore, data collection methods must ensure the availability of relevant and accurate data, while data cleaning and preprocessing techniques are essential for removing inconsistencies and errors that could skew the results of predictive modeling.
Predictive Modeling Techniques
Predictive modeling encompasses a variety of techniques, including regression analysis and machine learning algorithms such as decision trees, random forests, and neural networks. These techniques analyze historical data to identify patterns and relationships that can be used to make predictions about future outcomes.
Applications of Predictive Analytics
Predictive analytics finds applications across a wide range of industries, including finance, marketing, healthcare, and manufacturing. From predicting customer churn to forecasting demand for products and services, predictive analytics empowers businesses to make proactive decisions and optimize their operations.
Benefits of Predictive Analytics
The benefits of predictive analytics are manifold. By anticipating trends and future events, businesses can gain a competitive edge, optimize resource allocation, and enhance customer experiences. Predictive analytics also enables organizations to mitigate risks and capitalize on opportunities for growth and innovation.
Challenges and Limitations
Despite its potential, predictive analytics faces challenges related to data privacy, ethics, and implementation. Ensuring the responsible use of data, addressing bias and fairness in predictive modeling, and overcoming technical and organizational barriers are essential for successful predictive analytics initiatives.
Future Trends in Predictive Analytics
The future of predictive analytics holds promise, with advancements in artificial intelligence, machine learning, and big data analytics driving innovation in predictive modeling techniques. Integration with emerging technologies like the Internet of Things (IoT) and blockchain further expands the possibilities for predictive analytics applications.
Case Studies
Real-world examples illustrate the transformative impact of predictive analytics across industries. From financial institutions leveraging predictive analytics to detect fraudulent transactions to e-commerce companies using predictive modeling to personalize recommendations for customers, case studies demonstrate the tangible benefits of predictive analytics in driving business success.
Best Practices
Effective predictive analytics relies on sound methodologies and best practices. From selecting the appropriate modeling techniques to validating and refining predictive models, organizations must follow established guidelines to ensure the accuracy and reliability of predictive analytics results.
Ethical Considerations
Ethical considerations are paramount in predictive analytics, particularly concerning data privacy, transparency, and fairness. Organizations must prioritize ethical principles and adhere to regulations and industry standards to maintain trust and integrity in their predictive analytics practices.
Building a Predictive Analytics Culture
Building a culture that embraces predictive analytics is essential for maximizing its value in organizations. By fostering a data-driven mindset, providing training and resources for employees, and integrating predictive analytics into decision-making processes, businesses can create a conducive environment for leveraging predictive insights effectively.
Measuring Success
Measuring the success of predictive analytics initiatives requires defining key performance indicators (KPIs) that align with business objectives. From tracking the accuracy of predictive models to monitoring the impact of predictive analytics on business outcomes, organizations must establish metrics to evaluate the effectiveness of their predictive analytics efforts.
Conclusion
In conclusion, predictive analytics represents a paradigm shift in how businesses approach decision-making in the digital age. By harnessing the power of data and advanced analytics techniques, organizations can anticipate trends, mitigate risks, and capitalize on opportunities for growth and innovation. As the digital landscape continues to evolve, predictive analytics will remain a cornerstone of success for forward-thinking businesses.
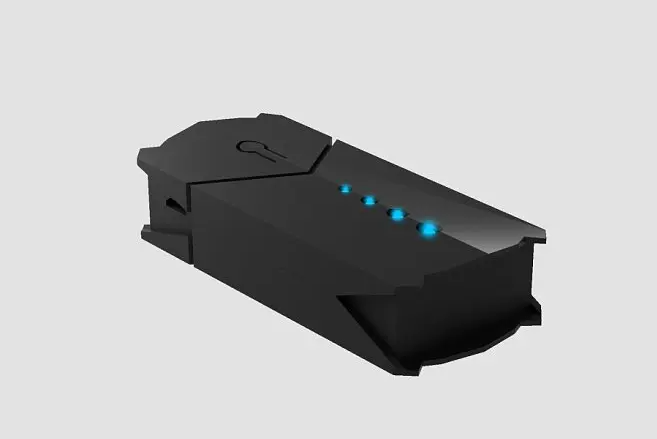
FAQs After The Conclusion
- What is predictive analytics?Predictive analytics is the use of data, statistical algorithms, and machine learning techniques to forecast future outcomes based on historical data.
- What are the benefits of predictive analytics?Predictive analytics enables businesses to anticipate trends, optimize resource allocation, enhance customer experiences, and mitigate risks.
- What challenges does predictive analytics face?Challenges in predictive analytics include data privacy concerns, ethical considerations, and technical and organizational barriers to implementation.
- What are some future trends in predictive analytics?Future trends in predictive analytics include advancements in artificial intelligence, machine learning, and integration with emerging technologies like IoT and blockchain.
- How can organizations build a predictive analytics culture?Organizations can build a predictive analytics culture by fostering a data-driven mindset, providing training for employees, and integrating predictive analytics into decision-making processes.
- What ethical considerations are associated with predictive analytics?Ethical considerations in predictive analytics include ensuring data privacy, transparency, and fairness in modeling and decision-making processes.
- What are some best practices for predictive analytics?Best practices for predictive analytics include selecting appropriate modeling techniques, validating predictive models, and prioritizing ethical principles in analysis.
- How can organizations measure the success of predictive analytics initiatives?Organizations can measure the success of predictive analytics initiatives by defining KPIs aligned with business objectives and tracking metrics related to predictive model accuracy and impact on business outcomes.