Introduction
In the digital age, the sheer volume of data generated presents both opportunities and challenges for businesses. To unlock the value hidden within this data, organizations are increasingly turning to artificial intelligence (AI) and machine learning. These advanced technologies play a pivotal role in extracting valuable insights from digital data, enabling businesses to make informed decisions and gain a competitive edge.
Fundamentals of AI and Machine Learning
AI refers to the simulation of human intelligence processes by machines, including learning, reasoning, and self-correction. Machine learning, a subset of AI, focuses on the development of algorithms that allow computers to learn from and make predictions based on data. Key concepts in machine learning include algorithms, which are the mathematical models used to analyze data, training data, which is used to train the algorithms, and models, which are the output of the training process.
Applications in Digital Insights Extraction
AI and machine learning have diverse applications in extracting insights from digital data. In marketing, for example, these technologies are used to analyze customer behavior and preferences, allowing businesses to tailor their marketing strategies accordingly. In finance, AI-powered algorithms are employed for fraud detection and risk assessment. Similarly, in healthcare, machine learning algorithms can analyze medical imaging data to assist in diagnosis and treatment decisions.
Data Collection and Preparation
High-quality data is essential for the success of AI and machine learning algorithms. Organizations must employ robust strategies for collecting and preprocessing data to ensure its accuracy and relevance. This involves cleaning the data to remove errors and inconsistencies, as well as transforming it into a format suitable for analysis.
Feature Engineering and Selection
Feature engineering involves selecting and transforming raw data into meaningful features that can be used by machine learning algorithms. This process is crucial for improving the performance of models and extracting relevant insights from the data. Techniques such as dimensionality reduction and feature scaling are commonly used to streamline the feature selection process.
Model Training and Evaluation
Once the data is prepared and features are engineered, machine learning models are trained using supervised or unsupervised learning techniques. Supervised learning involves training the model on labeled data, while unsupervised learning relies on unlabeled data. Models are evaluated using various metrics such as accuracy, precision, and recall to assess their performance and identify areas for improvement.
Predictive Analytics
Predictive analytics, enabled by AI and machine learning, involves using historical data to make predictions about future events or trends. Predictive models can be used in various domains, including sales forecasting, demand planning, and churn prediction. By leveraging predictive analytics, businesses can anticipate market trends and customer behavior, enabling proactive decision-making.
Pattern Recognition and Anomaly Detection
AI and machine learning are adept at recognizing patterns and detecting anomalies in digital data. Pattern recognition algorithms can identify recurring trends or patterns, while anomaly detection algorithms can flag unusual or suspicious data points. These capabilities are invaluable for detecting fraud, identifying cybersecurity threats, and monitoring system performance.
Natural Language Processing (NLP)
NLP is a branch of AI that focuses on enabling computers to understand, interpret, and generate human language. NLP techniques are widely used for analyzing textual data from sources such as social media, customer reviews, and emails. Applications of NLP include sentiment analysis, chatbots, and text summarization, allowing businesses to extract insights from unstructured text data.
Computer Vision
Computer vision involves teaching computers to interpret and analyze visual information from images or videos. AI-powered computer vision algorithms can recognize objects, extract information from images, and even understand human gestures and emotions. Applications of computer vision range from autonomous vehicles and medical imaging to surveillance and augmented reality.
Ethical Considerations
While AI and machine learning offer tremendous potential, they also raise ethical concerns related to privacy, fairness, and accountability. Organizations must ensure that their use of these technologies is transparent, ethical, and aligned with regulatory requirements. Privacy-preserving techniques, bias mitigation strategies, and robust governance frameworks are essential for addressing these ethical considerations.
Future Trends
The future of AI and machine learning in extracting digital insights is promising, with continued advancements expected in areas such as deep learning, reinforcement learning, and automated machine learning. Additionally, the integration of AI into various industries and the proliferation of AI-powered solutions will reshape business processes and workflows, driving innovation and efficiency.
Challenges and Limitations
Despite their benefits, AI and machine learning also face challenges and limitations. These include data quality issues, algorithmic bias, and the need for skilled talent. Overcoming these challenges requires a holistic approach that addresses technical, ethical, and organizational factors.
Case Studies
Several organizations have successfully leveraged AI and machine learning to extract valuable insights from digital data. For example, a retail company used predictive analytics to optimize inventory management and improve sales forecasting, resulting in increased efficiency and profitability. Similarly, a healthcare provider implemented NLP techniques to analyze patient feedback and identify areas for improvement in service delivery.
Conclusion
In conclusion, AI and machine learning play a crucial role in extracting digital insights that drive business growth and innovation. By harnessing the power of these technologies, organizations can unlock the potential of their data, gain actionable insights, and stay ahead of the competition in today’s digital landscape.
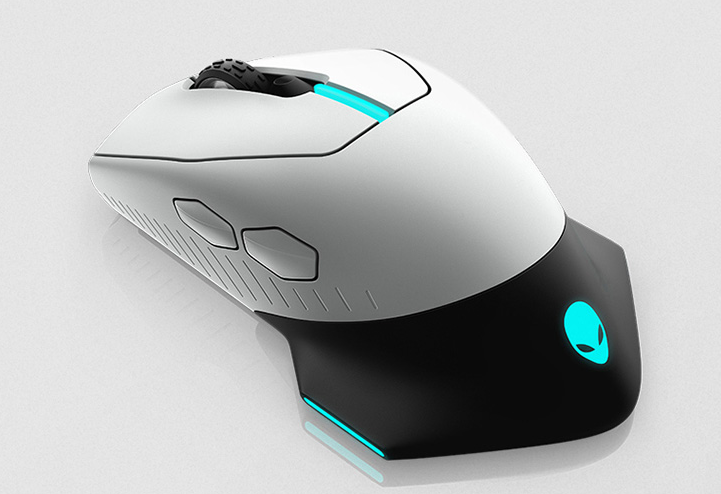
FAQs After The Conclusion
- What is the role of AI and machine learning in digital insights extraction?AI and machine learning are used to analyze digital data and extract valuable insights that inform business decisions and strategies.
- What are some common applications of AI and machine learning in digital insights extraction?Applications include predictive analytics, pattern recognition, natural language processing, and computer vision.
- Why is data collection and preparation important in AI and machine learning?High-quality data is essential for training accurate and reliable machine learning models. Data must be collected and prepared carefully to ensure its quality and relevance.
- How are machine learning models trained and evaluated?Models are trained using supervised or unsupervised learning techniques and evaluated using metrics such as accuracy, precision, and recall.
- What are some ethical considerations related to AI and machine learning?Ethical considerations include privacy, fairness, bias, and accountability. Organizations must ensure that their use of AI is transparent, ethical, and aligned with regulatory requirements.
- What are some future trends in AI and machine learning?Future trends include advancements in deep learning, reinforcement learning, and automated machine learning, as well as increased integration of AI into various industries and domains.
- What are some common challenges and limitations of AI and machine learning?Challenges include data quality issues, algorithmic bias, and the need for skilled talent. Overcoming these challenges requires a holistic approach that addresses technical, ethical, and organizational factors.
- Can you provide examples of organizations using AI and machine learning for digital insights extraction?Examples include retail companies using predictive analytics for inventory management, healthcare providers using NLP for patient feedback analysis, and financial institutions using machine learning for fraud detection.